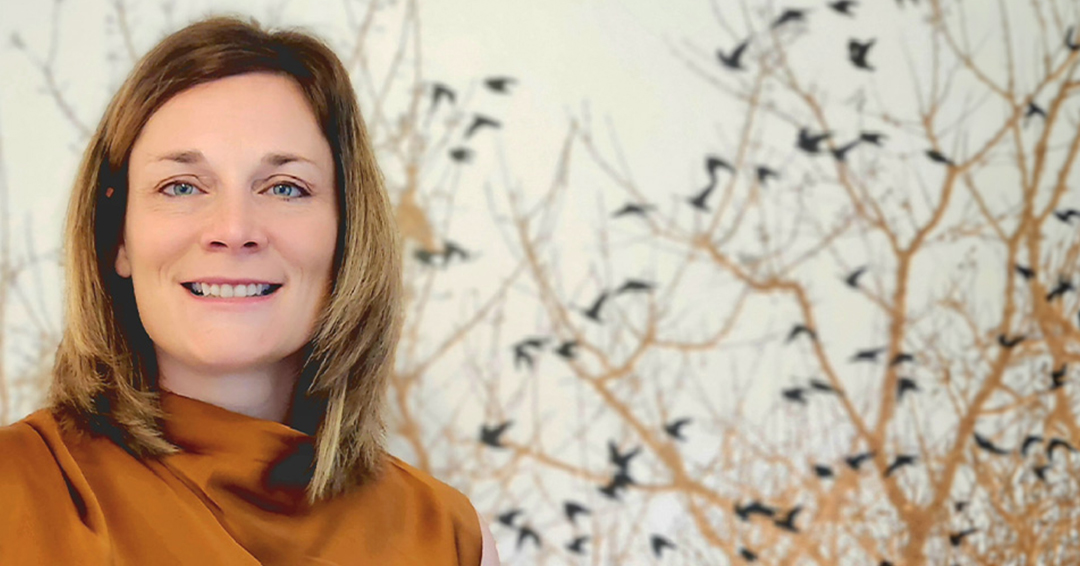
Corina Nagy, PhD, is an Assistant Professor in the department of Psychiatry at McGill University and member of the McGill Group for Suicide Studies. Here, she tells us about the promises and the challenges of single-cell genomics research in exploring the underlying mechanisms of depression.
Tell us about your research.
My lab has two complementary focuses revolving around a central topic, which is depression and the interaction with sex. The sex of the individuals plays a big role both in the presentation and the prevalence of depression, which has historically been overlooked. Even when accounting for issues related to reporting or diagnostic approaches, females are twice, if not more, as likely to develop depression, and this holds true globally. There is even molecular evidence suggesting differences in brain mechanism. For example, male patients respond better to tricyclic antidepressants, whereas females respond better to selective serotonin reuptake inhibitors (SSRIs). This led me to try and understand how this molecular framework can lead to the same phenotype (depression) through a different pathway. To understand if these differences come from changes in proportions of cells or the gene programming, my research focuses on understanding this at single-cell levels.
The other aspect of our lab’s research is to connect the central and peripheral systems to each other. I’m focusing on exercise as an adjunctive therapy for the treatment of depression, and the mechanism through which exercise contributes to both the prevention and amelioration of depression symptomology. But that’s tangential to my work related to the Ludmer Centre, so I will focus on the single-cell aspect of my research. Eventually, as technology continues to evolve, the two will merge.
Is this research done primarily in human tissue?
In both instances, we’re working with human and animal brain tissue. The difference in the prevalence of depression between males and females only occurs at puberty, which is a difficult stage to obtain post-mortem tissue from. One advantage of using animal models is that my research is done in a pseudo longitudinal manner, which requires samples at many different time points. Additionally, we use a mouse model called the “four core genotype”, which allows us to decouple the effects of hormones from genes. This is relevant during puberty when hormonal surges start the sexual maturation process.
How can single-cell genomic enhance our understanding of depression?
Treatments typically target the serotonergic system, and we know that antidepressants are effective in about 50% of people, and a large proportion remain treatment resistant. Other lines of pharmacotherapy involve ketamine and psilocybin, which do not affect the typical serotonergic targets, if at all. This suggests that serotonin is not the only factor at play. There can be an increase in inflammation, or a dysregulation of the excitatory/inhibitory (EI) balance. Most likely, it’s not any one thing in an individual at any time. This is particularly true when considering the symptomology can be on the opposite ends of the spectrum, for example hypersomnia or insomnia.
Using single-cell omics will allow us to take cell types and changes in cell types and to generate self-organizing maps or other data driven approaches to tell us how symptom clusters influence cells. This fine-grained resolution will help us develop more targeted interventions, instead of administering treatment affecting every neuron in a generic fashion.
So far in your research, have you identified any sex difference in cell expression?
We found a very strong and pronounced signal from microglia cells in females. This signal is also present in males, but it’s less pronounced. This could suggest that some of these symptoms may be regulated by microglia in the brain of females versus males. Because this is done in post-mortem human tissue, these findings are very correlational. But there’s a lot of research showing that early on in development, microglia are one of the cells that respond strongly to hormonal surges and can change their composition and their interactions with other cell types. If microglia can be so influenced by sex as a biological variable and their particular hormonal milieu, then perhaps this is a framework that leads to vulnerability in females.
If this work is done in post-mortem tissue, then one assumption could be that these individuals were affected by very severe depression, which has led to suicide. Can these findings be generalizable to the full spectrum of depression?
It’s possible that what we’re finding in the brain of individuals in this very severe state of depression may not apply to people who have a milder form of depression, or depression with very different symptomology, such as geriatric depression, or postpartum depression. And though we use animal models, a mouse never develops depression in the wild. There are limitations in both animal and human models using post-mortem tissue and we have to remain cautious in our generalizations of the data.
The Douglas Bell Canada Brain Bank (DBCBB) includes primarily brains from Caucasian individuals, which also raises the question about generalizability. We talked about sex, but gender also has an important influence on biological and physiological outcomes. Studies show that gender influences positive outcome to treatments and reporting. Brain banks around the world have not historically documented gender and moving forward, we need to include this in our data. Ideally, we would do this on larger scale to incorporate different phenotypes and the different phases of depression, and to include more diverse backgrounds and the full spectrum of depression and its symptomology.
How does open science impact your work? How do you benefit from it?
How do you contribute to it?
Open science means that all this data is out there and is available for different researchers to use. It also requires us to make sure we’ve analyzed our data in the same way. Single-cell genomics has been around for roughly a decade but it’s still in its infancy. It’s so complex and requires so much analysis and computation which we are developing as we move forward. First, we needed to generate the data, and now we need to analyze it in a consistent and standardized way. All our data is available, and most researchers typically do the same. Analytical tools are open source as well to ensure that we are using the same techniques and computing languages, and that we are labelling a feature the same way. When working with other brain banks, we have to make sure that our neuroanatomists are processing the tissue in the same way. Therefore, it’s very important that all of the tools and the data generated become available to ensure validity and reproducibility. It is very exciting to think of a future where we can generate all this data from around the world and bring it all together. The possibilities are endless.
What potential implications do you see for personalized treatment of depression based on your findings?
With current treatments, we don’t know the full mechanism of action. With SSRIs for example, we know that they inhibit serotonin reuptake, but there are other non-documented downstream effects which lead to side effects. For example, Tricyclics administration can lead to sedation and nausea and increase appetite. Often, side effects are what lead to noncompliance with the treatment.
So if we have a very targeted molecule that we know will be effective, then we’re more likely to treat the pathology with minimal side effects.
We could also correlate the changes in gene transcription of a given cell type to different symptomology and find molecules that would be effective in the treatment of a certain manifestation of depression. That’s ultimately what we would want to accomplish.
What are you most excited about with regards to your work in the next couple years?
So far, we discussed in broad strokes the pseudo-longitudinal profiling to track hormonal changes at different time points. This has the potential to tell us about cell-specific dynamics over time in males and females. We will be able to actually plot how these cell programs change and how that influences the cell’s microenvironment as well. For example, if microglia cells are undergoing changes in females after puberty, then one would anticipate that the cells that it surrounds and communicates with are going to be influenced. With spatial transcriptomic, we have the ability to look at cell neighborhoods to give us a dynamic, rather than a static, view of a cell. This is very ambitious because there are so many different cell types and different brain regions, and all of them are important. But we’re getting closer to narrowing down key time points. We want to see how dynamic the changes in this period are, but also the patterns that are maintained into adulthood. I’m really excited about having this dynamic view, rather than just a cross-sectional period in an individual.